Understanding the Basics of Gene Ontology (GO)
Gene Ontology (GO) provides a standardized framework for annotating genes and their products, allowing for the consistent description of gene functions across species. GO facilitates the comparison of gene functions by using a controlled vocabulary and a hierarchical structure, helping researchers categorize genes into three main ontologies: Biological Process (BP), Molecular Function (MF), and Cellular Component (CC).
Biological Process (BP)
The Biological Process ontology encompasses the series of molecular events that result in a specific biological outcome, such as cell division or metabolism. BP terms describe overarching biological activities involving multiple gene products, providing insight into large-scale physiological events. These terms are crucial for understanding how genes contribute to fundamental processes in the cell and organism, helping to link specific genes to cellular functions.
Molecular Function (MF)
The Molecular Function ontology describes the biochemical activities of gene products, such as enzyme catalysis or molecular binding. MF terms focus on the direct molecular activities of proteins or RNA molecules, such as "ATPase activity" or "DNA binding." These annotations provide essential details about how gene products interact with other molecules, enabling the cellular machinery to function.
Cellular Component (CC)
The Cellular Component ontology specifies the location within the cell where gene products are active, such as the nucleus or mitochondrion. CC terms describe where in the cell a gene product performs its function, helping to elucidate the spatial organization of cellular processes. These annotations are crucial for understanding the context in which genes operate and interact within the cellular environment.
Hierarchical Structure of GO
GO terms are organized hierarchically, with parent terms representing broad categories and child terms offering more specific descriptions. This structure allows researchers to navigate from general biological concepts to detailed functional annotations. The hierarchy ensures consistency in the application of terms, making it easier to map gene functions across different organisms and studies.
The Process of GO Annotation
Gene Ontology (GO) annotation involves assigning GO terms to genes and their products to describe their biological roles, molecular functions, and cellular locations. This process helps researchers understand the functional relevance of genes and their contributions to cellular activities. The GO annotation process can be carried out through various methods, each depending on the type of data available and the accuracy required.
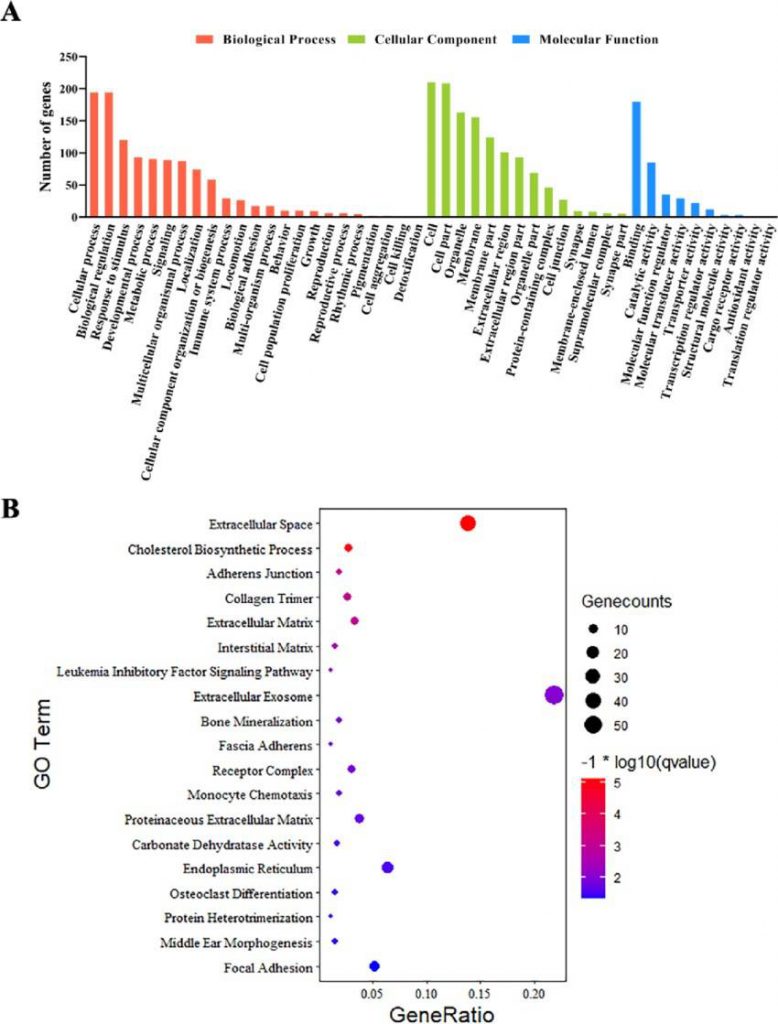
GO annotation and enrichment analysis. (A) GO annotation results for the total number of DEGs (Fisher's exact test, Q-value < 0.05). (B) GO enrichment analysis. The top 19 pathways with minimum Q values (Q-value < 0.05) have been shown (Yue et al., 2023).
Methods of GO Annotation
GO annotation can be performed using three primary approaches: manual curation, computational prediction, and automated annotation.
- Manual Curation: This method involves experts reviewing literature and experimental data to assign GO terms to genes. Manual curation ensures the highest accuracy since it is based on peer-reviewed and experimentally validated information. Curators rely on direct experimental evidence, such as gene knockout studies, protein assays, and genetic interaction data, to link genes with specific GO terms.
- Computational Prediction: Computational methods predict GO terms for genes based on sequence homology and functional domain analysis. When a gene shares significant sequence similarity with a well-characterized gene, it may be annotated with similar GO terms. Tools such as BLAST and InterProScan can identify conserved domains and sequences, facilitating the assignment of relevant GO terms without direct experimental data.
- Automated Annotation: This approach uses algorithms and bioinformatics tools to automatically assign GO terms based on large-scale data. Automated annotation is often used for annotating newly sequenced genomes or genes lacking experimental data. While it is less precise than manual curation, it allows for rapid annotation of vast numbers of genes. This method is commonly employed by large-scale databases like UniProt and Ensembl.
Sources of GO Annotations
GO annotations come from various sources, ensuring broad coverage and accuracy. These include:
- Gene Ontology Consortium: The Gene Ontology Consortium provides a central, authoritative source for GO terms and annotations, ensuring consistency and standardization across different organisms and research communities.
- Public Databases: Online resources such as Ensembl, UniProt, and NCBI provide GO annotations that are derived from both experimental evidence and computational predictions. These databases frequently update their annotations as new research findings become available.
- Literature: Curators extract GO terms from primary research articles, ensuring that the annotations reflect the latest experimental evidence. These annotations are particularly important when a new function or biological role for a gene is discovered.
Types of Evidence Supporting GO Annotation
GO annotations are supported by different types of evidence, which determine their reliability. The main categories of evidence include:
- Experimental Evidence: This is the most reliable form of evidence and comes from direct experimental studies. Techniques such as gene knockouts, overexpression studies, and protein interaction assays are used to assign GO terms. For instance, if knocking out a gene leads to a disruption in a specific biological process, the gene can be annotated with the corresponding GO terms.
- Computational Evidence: Computational evidence is derived from sequence similarity to well-characterized genes, protein domains, or known functional motifs. Tools like BLAST and InterPro can be used to predict functional associations based on sequence alignment. While less direct than experimental evidence, computational evidence helps annotate genes with minimal experimental data.
- Curated Evidence: Curated evidence involves the manual review of literature and experimental results. Curators assign GO terms based on previously published research, offering a high degree of accuracy and validation. Curated annotations are often more detailed, incorporating functional relationships between genes, pathways, and biological contexts.
- Inference from Electronic Annotation (IEA): Electronic annotation involves using algorithms to infer GO terms based on sequence homology to genes with known functions. Though not experimentally validated, IEA is a useful tool for annotating large numbers of genes when experimental data is scarce.
GO Annotation Tools
Several tools and resources support GO annotation by automating or facilitating the process. Some of the widely used tools include:
- Blast2GO: This tool enables the assignment of GO terms to gene sequences by integrating sequence similarity data with functional annotations. It supports both the prediction of molecular function and the assignment of biological process and cellular component terms.
- QuickGO: A web-based tool that allows users to explore and visualize GO annotations for specific genes. QuickGO provides a detailed overview of gene functions, highlighting the associated GO terms and their relationships.
- DAVID: The Database for Annotation, Visualization, and Integrated Discovery allows for functional annotation and enrichment analysis, making it easier to interpret large sets of gene expression data in the context of GO terms.
- PANTHER: This tool is used for protein classification and GO term assignment, providing insight into gene function through pathway analysis and molecular classification.
Types of GO Annotation Evidence
GO annotations are supported by different types of evidence that determine their accuracy and reliability. These evidences provide varying levels of confidence in the functional roles assigned to gene products. The main types of evidence used for GO annotation are experimental evidence, computational evidence, curated evidence, and inference from electronic annotation (IEA).
Experimental Evidence
Experimental evidence is considered the most reliable form of evidence for GO annotation. It involves direct laboratory studies and results that validate the function of a gene product. This evidence comes from a wide range of experimental approaches, such as gene knockouts, protein assays, and gene expression analysis. For example, if the deletion of a gene disrupts a biological process, it can be annotated with the corresponding GO terms related to that process.
Experimental evidence provides a high level of confidence in the accuracy of the assigned GO terms because it is based on actual observations and experimental data. This type of evidence is used when specific, experimentally validated functions are linked to a gene, such as enzyme activity, binding interactions, or participation in a biological pathway.
Computational Evidence
Computational evidence is based on the predicted functional associations of genes, often derived from sequence similarity or conserved protein domains. When a gene shares significant sequence similarity with a functionally annotated gene, it can be assigned similar GO terms. Tools like BLAST and InterProScan are commonly used to identify functional domains in a gene sequence that have been previously associated with specific biological processes, molecular functions, or cellular components.
Although computational evidence is less direct than experimental evidence, it plays a crucial role in annotating genes that lack experimental data. It enables researchers to predict gene functions based on homology to well-characterized genes, providing valuable information when experimental validation is not available. However, computational evidence should be used cautiously and often serves as supplementary data to experimental evidence.
Curated Evidence
Curated evidence refers to annotations derived from expert manual review of scientific literature and experimental results. Curators manually assign GO terms based on published research, ensuring that the annotations reflect the latest experimental findings. Curated evidence provides a high level of accuracy because it involves human expertise in interpreting experimental data.
This type of evidence is essential for the annotation of genes that have well-documented functions in the literature but may lack direct experimental data in public databases. Curators compile and synthesize findings from multiple studies to generate comprehensive and precise GO annotations. Curated evidence is also vital for updating and refining annotations as new data becomes available.
Inference from Electronic Annotation (IEA)
Inference from electronic annotation (IEA) is a form of indirect evidence that relies on automated tools to infer GO terms for genes based on sequence homology or computational predictions. In IEA, GO terms are assigned to genes by algorithms that identify sequence similarities with genes whose functions are already known. Although this method does not rely on experimental data, it can be useful for rapidly annotating large datasets when experimental evidence is lacking.
IEA is typically considered lower in confidence compared to experimental or curated evidence. It is often used for genes that have no experimental validation and may be based on weak or broad sequence similarities. IEA annotations can help researchers quickly assign functional information, but they may need further refinement as more experimental data becomes available.
Applications of GO Annotation
Functional Analysis
GO annotation allows researchers to categorize genes based on their biological processes, molecular functions, and cellular components. This classification aids in understanding the role of specific genes in cellular systems. By annotating genes with relevant GO terms, scientists can identify genes involved in particular processes or activities, such as metabolic pathways, signal transduction, or protein synthesis. This enables a better understanding of how genes contribute to complex cellular and organismal functions.
Pathway Analysis
GO annotations are essential for identifying and analyzing gene networks and biological pathways. Researchers can use GO terms to map genes to specific pathways, such as the cell cycle, apoptosis, or immune response. This approach helps identify functionally related genes that work together to perform coordinated actions. Pathway analysis using GO terms allows for the identification of potential regulatory mechanisms, interactions, and feedback loops within cellular systems, contributing to the understanding of disease mechanisms and cellular responses.
Comparative Genomics
In comparative genomics, GO annotation facilitates the comparison of gene functions across different species. By annotating genes with consistent GO terms, researchers can identify homologous genes and determine how gene functions have evolved. This is particularly valuable when studying species with incomplete or poorly annotated genomes, as GO terms allow for the prediction of gene functions based on sequence homology. GO annotations enable researchers to trace evolutionary relationships and assess the conservation or divergence of specific biological processes across species.
Disease Research
GO annotation is increasingly used in disease research to identify genes associated with specific diseases and to better understand disease mechanisms. By analyzing the GO terms of genes associated with particular diseases, researchers can uncover common biological pathways or processes implicated in the disease. For example, genes related to cancer may be found to share specific GO terms related to cell cycle regulation or apoptosis. GO annotations also help identify potential therapeutic targets by highlighting key genes involved in disease-related pathways.
Gene Set Enrichment Analysis (GSEA)
Gene set enrichment analysis (GSEA) is a statistical method used to identify biological pathways or processes that are overrepresented in a set of genes, often derived from gene expression data. GO annotations are integral to GSEA, allowing researchers to analyze gene expression datasets in the context of biological functions. By using GO terms, researchers can identify biological processes or molecular functions that are significantly enriched in different experimental conditions, helping to interpret the biological significance of gene expression changes.
High-Throughput Data Interpretation
With the advent of high-throughput technologies such as RNA-seq and microarrays, GO annotation has become indispensable for interpreting large-scale gene expression data. By annotating genes with GO terms, researchers can systematically categorize gene expression profiles and identify biologically relevant trends across conditions. GO annotation helps make sense of vast datasets by providing a framework for interpreting gene function in the context of experimental conditions.
Creative Proteomics provides GO annotation services for proteomics, metabolomics, and glycomics to help researchers better understand the functional roles of proteins, metabolites, and glycans. By applying GO, we assign relevant biological process, molecular function, and cellular component terms to these biomolecules, enabling deeper insights into their involvement in cellular and physiological processes. Our advanced computational tools and up-to-date databases ensure accurate and reliable GO annotations, supporting functional analysis, pathway mapping, and biomarker discovery across various omics studies.
Reference
Yue, Qiaoxian, et al. "Transcriptomic analysis reveals the molecular mechanisms underlying osteoclast differentiation in the estrogen-deficient pullets." Poultry Science 102.3 (2023): 102453.